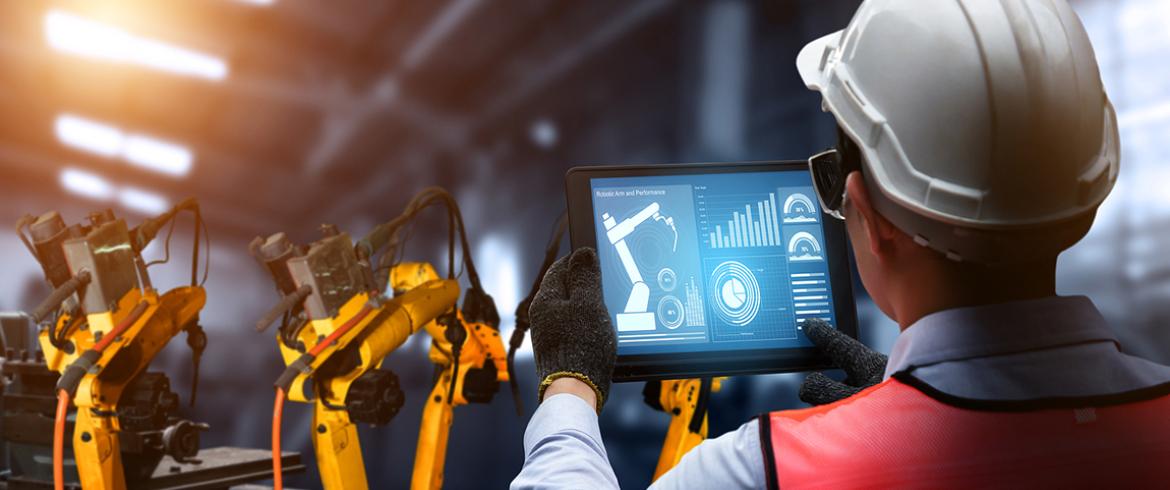
Researchers from the FAMU-FSU College of Engineering and Virginia Tech are collaborating to advance smart manufacturing and advanced material manufacturing using artificial intelligence. The novel technique may enhance performance by alleviating the uncertainties associated with operations.
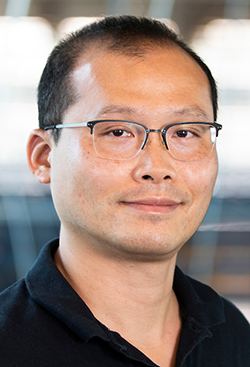
The work involves complex AI-enabled engineering systems such as smart factories and advanced manufacturing. Like the name implies, a smart factory is an interconnected network of machines, feedback systems, and computational capacity. This cyber-physical system analyzes its own data to drive automated processes and learn (improve) as it operates. But this now-commonplace technology isn’t without challenges.
“An issue that plagues many engineering systems involves jump behaviors when a system performance operation is suddenly disrupted,” said Chiwoo Park, an associate professor in industrial and manufacturing engineering at the college. “For example, in smart factories, as long as there is a regular load on the system, the performance and efficiency will be pretty good. However, if you have a demand surge over capacity, the system can fail and the performance can drop significantly.”
Park says identifying the jump behaviors helps in understanding the capacity. In addition to understanding how this issue affects smart factories, the team wants to improve jump behavior associated with advanced material manufacturing.
“Jump behaviors are also prevailing in the chemical processes and material synthesis in advanced materials manufacturing, due to phase changes and chemical equilibrium, “Park said. “We want to identify jump behavior to optimize the processes.”
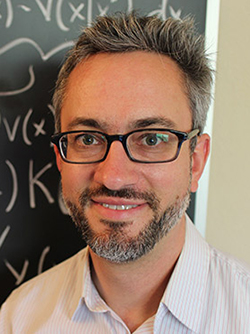
Park is working with Robert Gramacy, professor of statistics at Virginia Tech, on the project. The preliminary research work is featured in the Journal of Machine Learning Research.
The researchers are looking at major applications to the automated processes and internal factory logistics in smart factories. These factories might use a large fleet of material handling robots, overhead hoist transports and robotic lifts. Design parameters control operation and coordinate tasks. However, some variables can affect how well these operations are performed. Similar to jump behavior, unexpected disruptions can slow or halt production.
“We want to understand how performance changes when considering the variables involved in the process,” Park said. “By analyzing historical data involved, we can analyze the results and set up better parameters to help optimize processes.”
Each university team is working on specific algorithmic models to understand jump behaviors.
The FAMU-FSU Engineering researchers approach the problem using local adaptive models to predict system behavior. By taking historical, local data centered around a region of interest, they can quickly pinpoint data needed to solve the problem. The result is faster and can be more accurate than using the entire database.
“When it comes to local data features such as sudden jumps in system performances, using local data neighboring around regions of interest could be more effective,” Park said. “It is also more computationally expedient.”
The Virginia Tech researchers take the opposite approach. They want to capture a global view of system performance based on all historical data versus local data. The global view is less inefficient in locating globally local trends, like jump behavior. To supplement the lack of local adaptivity the new approach uses supplemental data specific to jump behavior when it builds a global view. Although the database is much larger the anomalies, they are looking for are narrow. They are specific enough to provide a model to help manufacturers better predict when a surge might occur.
The team believes the proposed research will have a profound impact on many scientific areas, not limited to smart manufacturing and advanced materials. It is also more broadly applicable to other application areas including adaptive sensing and prediction of heterogeneous systems and for quality engineering.
“The studies are complimentary,” Park said, “There is no one-size fits all solution in machine learning.”
The study is supported by two National Science Foundation grants through Florida State and Virginia Tech universities.
RELATED ARTICLES
Chiwoo Park publishes book Data Science for Nano Image Analysis