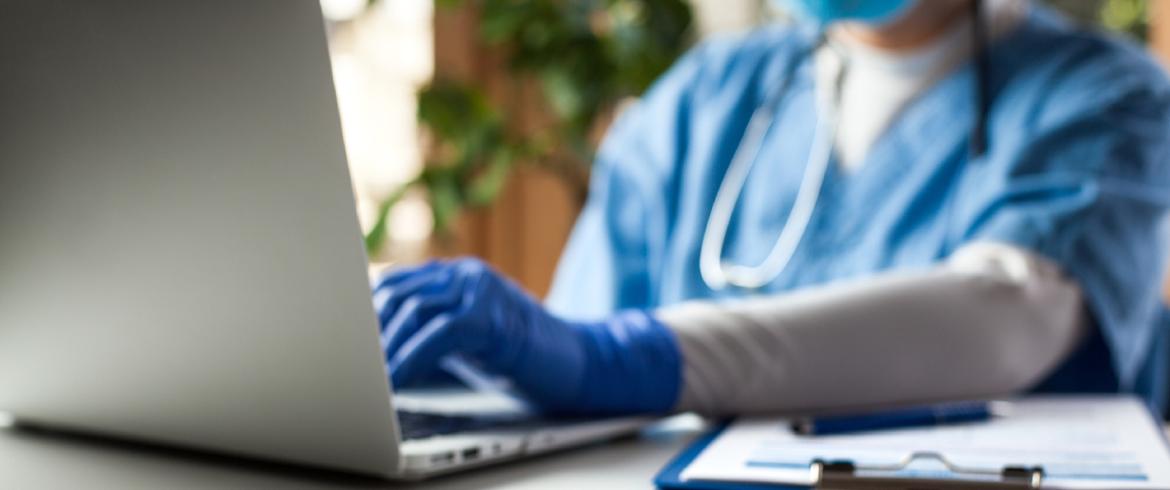
FAMU-FSU Engineering researchers have developed an advanced health surveillance tool to help improve response time to emerging pandemics, especially during natural disasters.
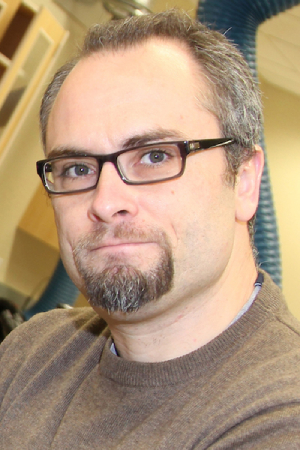
“One of the open research challenges in disease surveillance is how to make the detection algorithm sensitive, or adaptive, to the geographical size of potential outbreaks,” Rupert Giroux, a researcher on the team, said. “Having a detection algorithm that is sensitive to realistic outbreak scenarios gives health professionals an upper hand to be able to detect and locate outbreaks more quickly and devise effective disease containment measures.”
Giroux, a safety data coordinator for the Florida Department of Transportation who received his Ph.D. at the college, and Arda Vanli, an associate professor of industrial and manufacturing engineering, have been able to do just that with a targeted modeling method that efficiently models and monitors integer-value time series data. This type of data is commonly used to track everything from road safety to public health.
“Two of the critical questions that authorities want to answer are based on the Covid-19 cases that we have experienced so far,” Vanli said. “They want to know what caused the past outbreaks and where the next one might occur. The faster we can determine this, the sooner it can be contained.”
One characteristic of the Covid-19 pandemic is that it occurs in waves. Successive waves of outbreaks happen as new variants replace older ones. Researchers can use this new modeling method to utilize the information from each wave and determine the baseline error margins for the next wave.
“Public health authorities use monitoring systems to track infectious diseases and help determine where and when outbreaks might occur,” Vanli said. “However, it is a challenging and time-consuming task to manually process and interpret the massive amounts of heterogeneous data coming from all 67 Florida counties.”
The researchers created the innovative statistical modeling method to analyze large amounts of data in an adaptive and automated way. The method adapts to the size of a potential outbreak experienced in an area and requires less manual input than traditional methods. It allows public health authorities to more accurately and quickly determine where new geographically-concentrated cases might occur.
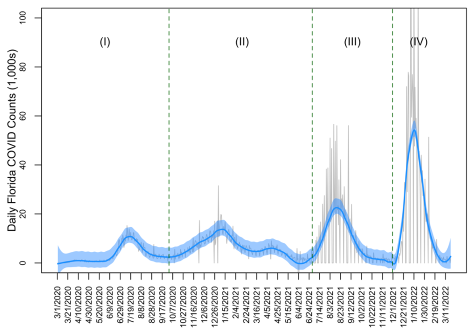
The Florida Department of Health built an Electronic Surveillance System for the Early Notification of Community-based Epidemics, called ESSENCE-FL, and collects near real-time pre-diagnostic data from participating hospitals and urgent care centers in Florida. With this new method, experts may be able to predict—and adapt—more quickly to emerging public health threats such as Covid-19.
Another area of interest to the researchers involves disaster response during a hurricane. Vanli is a researcher at the RIDER Center, dedicated to the resiliency of communities in a disaster. During hurricane season, a Florida hurricane shelter can be a hotspot for COVID-19 outbreaks.
“When a pandemic co-occurs with natural disasters, surveillance methods can prove to be effective in quickly detecting disease outbreaks in evacuation shelters,” Vanli said. “This can help emergency managers to implement non-congregate sheltering plans and protect our most vulnerable populations.”
The team uses a seasonal INGARCH time series model—a popular model for modeling a time series of counts—to estimate the baseline level of the infection data and a cumulative-sum detector to show the sum of data as it grows over time (similar to a running total). The process can quickly detect new and unobserved trends.
The research was recently documented in the journal Quality Engineering. The project was funded in part by the grant “Excellence in Research: Bending the Curve for Vulnerable Populations: A Data- Analytical and Socio-Technical Decision-Making Framework for Sheltering in Hurricane-Pandemics,” from National Science Foundation.