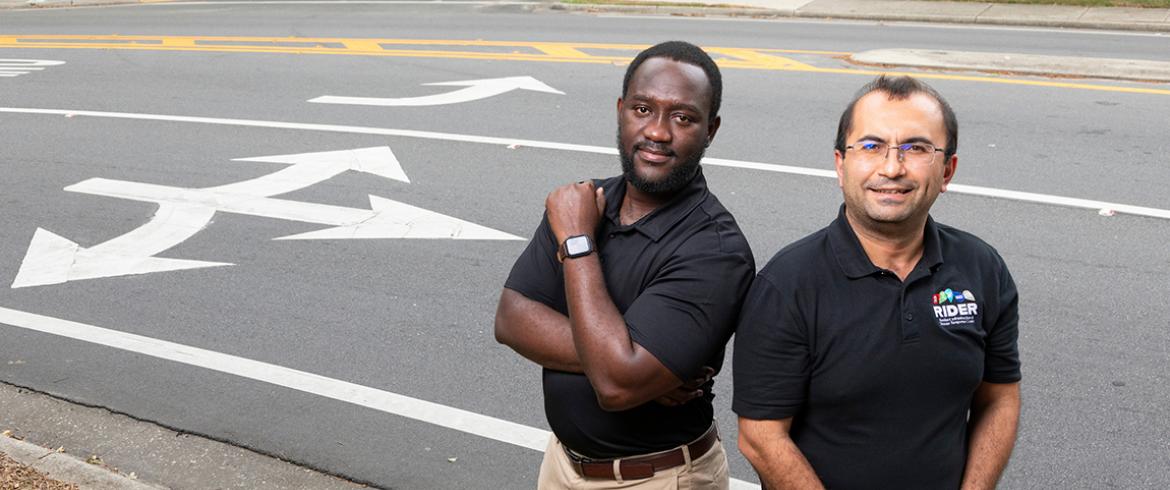
Richard Antwi (left) and Eren Ozguven, Ph.D., engineering researchers at the Resilient Infrastructure and Disaster Response Center (RIDER) pose near turn lane markings on Lake Bradford Road in Tallahassee, Florida on September 5, 2024. The team used AI to detect and extract turning lane features from aerial images. (Scott Holstein/FAMU-FSU College of Engineering)
Researchers at the FAMU-FSU College of Engineering have introduced a groundbreaking methodology that combines artificial intelligence (AI) and computer vision technology to transform how traffic agencies collect and analyze roadway geometry data.
A study published in Smart Cities details the new approach, which aims to streamline a traditionally labor-intensive process.
“Traditionally, gathering this data has been both labor-intensive and prone to human error,” said Richard Boadu Antwi, the lead researcher on the study. “It involves physically surveying roads and manually recording details.”
The new computer vision-based model promises higher accuracy by extracting valuable geospatial information about roadway features using aerial imagery and extensive data inventories. The research team employed a combination of image processing techniques and a YOLO (You Only Look Once) algorithm, focusing on high-resolution aerial images of Florida's public roadways.
“YOLO is an algorithm that helps computers interpret visual information the way humans do,” explained Eren Ozguven, a researcher involved in the study. “It helps us detect objects in images and frames of videos related to roadways.”
The study focused on roadways in Leon County, Florida, achieving an average accuracy rate of 87% at a 25% confidence threshold for detected features, such as left-turn, right-turn, and center lane markings. In practical terms, this approach successfully identified approximately 3,026 left turns, 1,210 right turns, and 200 center lane features across the county.
The study highlights that using computer vision techniques for roadway geometry extraction can significantly reduce the time, cost and errors associated with traditional manual inventory methods.
The precision of this data collection method is transformative for transportation agencies responsible for maintaining roadway safety and efficiency. The methodology allows for the identification of faded or missing markings, the comparison of turning lane positions with other roadway elements, and the analysis of intersection-related crashes. By integrating this data with crash and traffic information, policymakers and road users gain critical insights that enhance roadway safety.
The study highlights that using computer vision techniques for roadway geometry extraction can significantly reduce the time, cost and errors associated with traditional manual inventory methods.
However, the researchers also acknowledged certain limitations, particularly the challenge of canopy-covered roadways obstructing aerial view of lane markings. Despite these challenges, the study lays a foundation for future research, including integrating the model’s findings into established roadway geometry inventory datasets and expanding detection capabilities to encompass additional roadway features.
The study’s lead author, Antwi, was joined by fellow civil engineering faculty researchers Eren Ozguven, Ren Moses, and Maxim Dulebenets; doctoral student Samuel Takyi from the Resilient Infrastructure and Disaster Response (RIDER) Center; and Michael Kimollo and Thobias Sando from the University of North Florida, Jacksonville.
The research was funded by a grant from the Florida Department of Transportation (FDOT) and partially supported by the college’s USDOT University Transportation Center, Rural Equitable and Accessible Transportation (REAT) Center. It represents a significant advancement in roadway geometry data collection methodologies.
Looking ahead, the research team aims to broaden the scope of their work by integrating the extracted data with crash statistics, traffic patterns, and demographic information for a more comprehensive analysis of roadway safety.
For more information, read the full study in Smart Cities.
RELATED ARTICLES
Researchers Use Artificial Intelligence to Find Road Safety Issues in School Zones