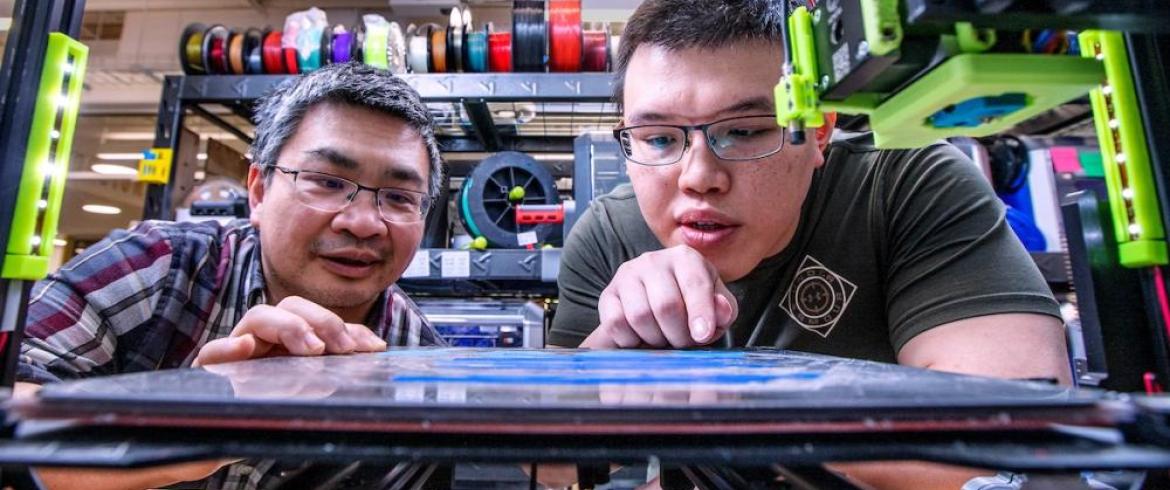
Hui Wang, left, associate professor of industrial engineering and An-Tsun Wei, a Ph.D. student, are the co-authors of a paper detailing how learning cloud data collected from interconnected 3D printers improves quality control and printing accuracy. (M Wallheiser/FAMU-FSU Engineering)
According to the on-demand manufacturing platform 3D Hubs 2021 3D Printing Trends Report, the global 3D printing market grew by 21 percent in 2020, despite the effects of a worldwide pandemic. This growing industry is accelerating to print everything from metal to biological materials. The race is on to optimize these processes for competitive advantage.
In a new National Science Foundation (NSF) study, researchers at the FAMU-FSU College of Engineering have developed a new technology that allows different printers to transfer learned experiences and lessons from each other. The process accelerates the printing control for extrusion-based additive manufacturing. The novel procedure may improve efficiency and quality for 3-D printing systems.
“Cloud manufacturing, along with the Internet of Things (IoT), is a technology that has emerged in recent decades,” said Hui Wang, associate professor at the FAMU-FSU College of Engineering. “The technology demonstrates that data generated from multiple production machines can be shared with each in a timely manner, and manufacturing can be enclosed as online services for meeting diverse market demands.”
Wang is the lead investigator from the Department of Industrial and Manufacturing Engineering (IME) at the college. He is working with a team of researchers to develop novel learning algorithms and process control methodologies. The technique utilizes cloud data to reduce printing defects and non-uniformity issues that may cause internal infill defects in the printed structures. Kinematic variations in the printer’s nozzle can cause variations and flaws.
Wang explains, “In the past, engineers did not have the motivation to connect machines together in the factory using this technology because the value of doing so was unclear. By developing novel machine learning algorithms we can demonstrate the value of cloud data.”
According to the researchers, the new application of learning cloud data collected from interconnected 3D printers improves quality control and printing accuracy. In the study, the researchers connected different printers on a cloud platform. The printers transferred experiences and shared knowledge. As a result, the lead time for preparing or calibrating the printer was reduced.
“In extrusion-based additive manufacturing, the material is selectively dispensed through a nozzle. Variation in the way the material is layered can cause defects and non-uniformity,” Wang said. “These problems impact the functions of printed structures, such as metamaterials, and the problem becomes more significant in collaborative printing systems where multiple printing nozzles co-create the same model.”
In recent decades, collaborative printing systems have become more favorable because they can significantly speed up the manufacturing process. But, it’s important to have a quantitative understanding of the effect printing process parameters have on the quality to guide the printing process control. Establishing a mathematical model to calibrate the printer parameters has been a challenge since multiple experiments are needed to collect data.
“We crafted a model that helps predict the infill pattern of non-uniformity that occurs,” An-Tsun Wei, a Ph.D. student in IME and co-author of the paper, said. “We can estimate geometric print quality and the related defects that might occur with the model. The information can be used to calculate adjustments needed in the input printing parameters to compensate for those errors.”
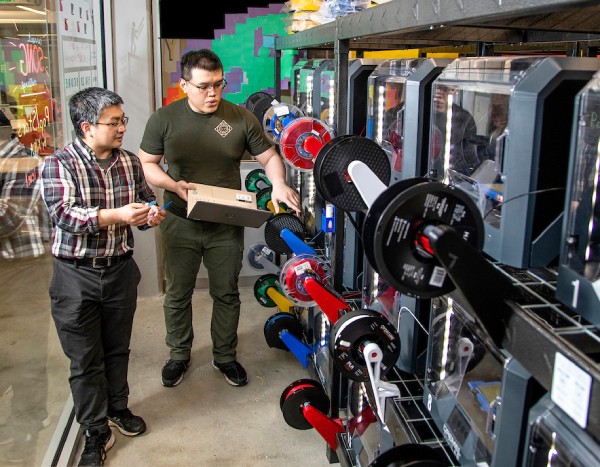
Traditional machine learning requires a lot of experimental data, which the industry may not have time to collect. What’s more, printers need to be quickly re-adjusted to cope with new printing tasks for flexible manufacturing. Transfer learning technology allows different printing processes to share experiences, thus accelerating this process. The research demonstrates the feasibility of using historically shared data from interconnected 3-D printers.
“The transfer learning algorithm established in this study allows different printers on a cloud platform to transfer experiences,” Wang said. “This reduced testing and measurements conducted during the print process and demonstrates significant improvement in predicting printing quality compared with traditional machine learning based on limited measurements on each process.”
The results are reported in a recent article on IEEE (Institute of Electrical and Electronics Engineers) Transactions on Automation Science and Engineering, a flagship journal in the field of using analytics to support system automation.
“With reduced testing, we can improve quality control faster and thereby quickly recalibrate the printing processes for meeting diverse market demands,” Wang said. “This is particularly suitable for mass production of personalized products, a manufacturing paradigm envisioned in the future.”
Wang calls this transfer learning a way to achieve “group intelligence” by which multiple learning agents (learners) collaborate to outperform a single learner. The technology can be applied to a variety of products using different materials.
The investigation is partially supported by two NSF grants totaling over $1.3 million. One engages the NSF’s Research Infrastructure for Science and Engineering program (RISE) and Research Experiences for Undergraduate (REU) at the High-Performance Materials Institute at the college. The platform aims to establish learning methodologies leading to a viable way to create high-performance lightweight composite structures in various size scales. The other NSF grant supports research on flexible manufacturing to meet diverse market demands.