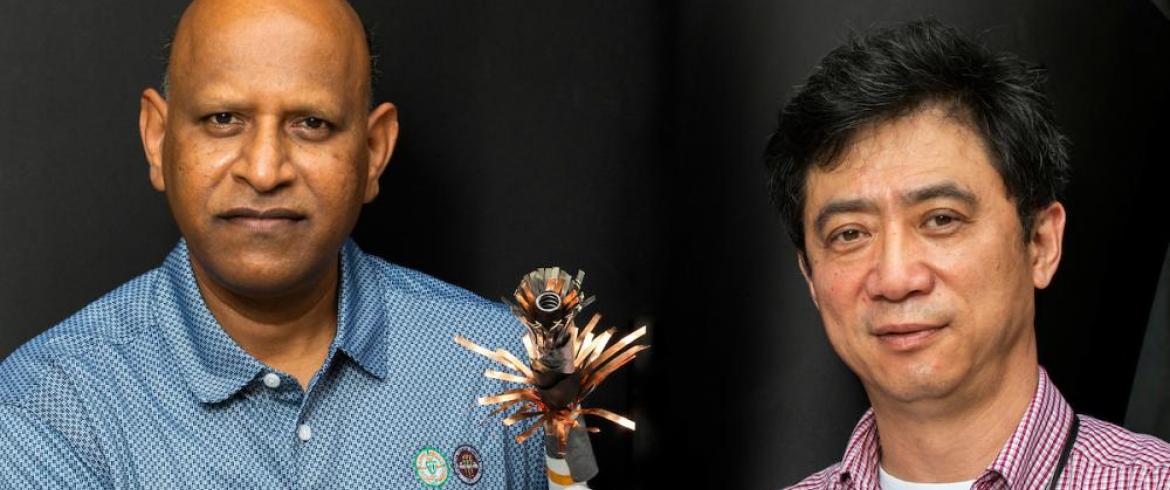
Professor Sastry Pamidi, Ph.D., left, Chair of the Department of Electrical and Computing Engineering at FAMU-FSU Engineering and Professor Chul Han Kim, Ph.D. with one of the HTS superconducting power cables they are developing for the U.S. Navy. (Photo: FAMU-FSU Engineering/M Wallheiser)
In a new study, engineering researchers are developing a novel machine learning tool that may increase the reliability of high-temperature superconductor (HTS) power systems. The method finds early indicators of series faults in HTS cables.
High-temperature superconductor power systems can carry 100 times more electricity than conventional wires. They are lightweight and require less space to power electric aircraft and ships.
Series faults occur when one or more conductors fail, creating an imbalance that can cause problems in the system. Detecting defects early greatly improves the life and safety of the cables and prevents catastrophic and costly damage.
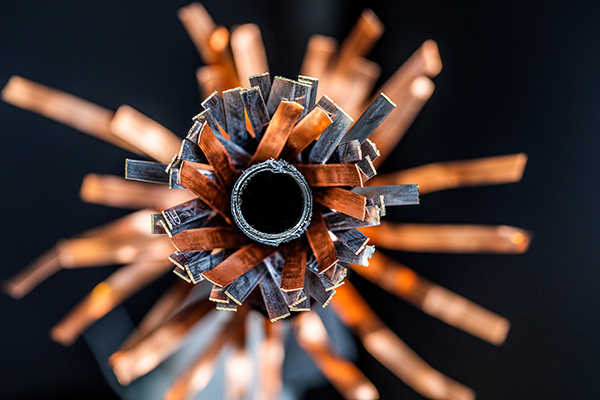
Sastry Pamidi, chair of the FAMU-FSU College of Engineering’s electrical and computer engineering department and the Associate Director of the Center for Advanced Power Systems (CAPS) at Florida State University is a lead researcher on the study that will help improve early fault detection.
“We’re collaborating with Dr. Lukas Graber’s group at Georgia Tech on multiple projects,” Pamidi said. “We are developing a machine learning tool to detect the magnetic signature of HTS cables in order to monitor its transmission characteristics.”
The magnetic signature of the cables allows the researchers to see the faults and is especially important when working with HTS cables. Superconducting cables developed at CAPS are used for U.S. Navy electric ship and aircraft technologies.
The project team includes Pamidi and members of his research staff, CAPS research faculty members Peter Cheetham and Chul Kim, along with researchers from the Georgia Institute of Technology, Mississippi State University, and the Korea Electric Power Corporation.
Machine learning is a form of artificial intelligence that enables a system to learn from data rather than through explicit programming. It is not a simple process. As the algorithms ingest training data, it is possible to produce more precise models based on that data.
“The machine learning tool we are working with can predict a series fault analysis to evaluate the effect of open circuits or asymmetrical line impedance conditions on the network,” Pamidi said.
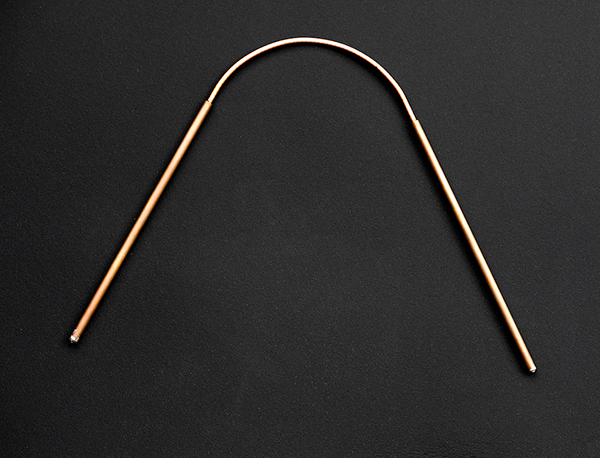
The researchers implemented a scattering parameter model for their study. This model, also called an S-parameter, describes the electrical behavior of linear electrical networks while undergoing various steady-state stimuli by electrical signals. The technique has the potential to enhance high-temperature superconductor power system reliability.
“We used a regression algorithm to predict outcomes using the Scikit-learn library,” Graber said.
The Scikit-learn is a free software machine learning library for the Python programming language.
The research is funded by the Office of Naval Research. The research was part of an ongoing long-term collaborative research between the Center for Advanced Power Systems and the Georgia Institute of Technology.
Their findings were recently published in the IEEE Transactions on Applied Superconductivity Journal.