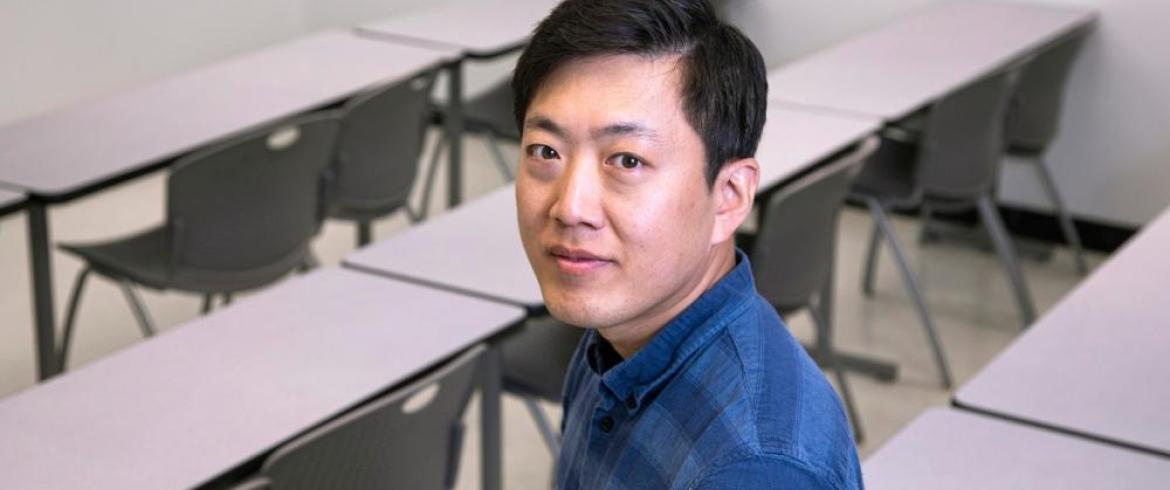
Jinyeong Moon, an assistant professor in electrical and computer engineering at the FAMU-FSU College of Engineering, recently co-authored an award-winning paper on the development of a ‘smart’ sensor node that can perform ‘predictive maintenance. (M Wallheiser/FAMU-FSU Engineering)
Jinyeong Moon, an assistant professor in electrical and computer engineering at the FAMU-FSU College of Engineering, won the Best Paper Award at the 31st International Conference on Field-Programmable Logic and Applications.
In partnership with researchers at the University of California, Irvine, Moon co-authored a paper for work that introduces a Long Short-Term Memory (LSTM) neural accelerator for low-power edge sensor nodes. The technology involves machine learning techniques for sensors.
The winning paper, “Eciton: Very Low-Power LSTM Neural Network Accelerator for Predictive Maintenance at the Edge,” is the work of a collaboration with Sang-Woo Jun, an assistant professor of computer science in the Donald Bren School of Information Sciences (ICS), and several ICS graduate students. A student from the Diamond Bar High School was also part of the project.
“Our research project is about developing a ‘smart’ sensor node that can perform ‘predictive maintenance,’” Moon said. “It is called a cyber-physical system or CPS and predicts the malfunction of a target system before it breaks down.”
The CPS acts as a tiny sentinel that monitors operating signatures including vibration or electrical signals. The group applied this method to two targets with known mechanical problems.
“Smart CPS sensor nodes can be used on electrical, mechanical and electromechanical systems that require maintenance over their lifetime,” Moon said. “Anything that requires a compressor, fan, electric motor or engine can benefit from our technology.”
Moon explained, “When the potential failure or malfunction is predicted early enough by our technology, then a lot of resources can be saved.
“A good example is discovering that you have a loose bolt and nut on the pool pump’s mount,” Moon said. “If you find a loose bolt early it takes ten seconds to retighten. However, if you let this ‘pathology’ fester to an extreme degree, the pool pump will come off its mount completely, vibrate and bounce around until it cracks the nearby pipes and damages electrical wirings.”
By automating the procedure, the Smart CPS can perform predictive maintenance continuously and detect problems before they occur. The CPS utilizes a Long Short Term Memory (LSTM) recurrent neural network and reduces the amount of computation needed. The neural network learns what a potential malfunction looks like in its early stage, and the memory eliminates the need for complex mathematical operations to identify the problem.
“The novelty of this work is that our CPS figures out the anomaly ‘onboard,’” Moon said. “Collecting data via sensors is usually not a demanding task for a CPS. But analyzing the data using mathematical operations requires a lot of computational power. That is where our technology excels.”
The new technology uses 17 milliwatts of power to find the malfunction. Compare this to a traditional CPS, which might use hundreds or thousands of milliwatts. The level of consumption is low enough to be powered by a tiny energy harvester without any battery or external power wiring.
Jun and Moon met while pursuing their doctorates at the Massachusetts Institute of Technology. The two have collaborated on research projects since 2019. Jun works with artificial intelligence, neural network and edge computing, while Moon works on the design and predictive maintenance of power electronics, energy harvesting and electric motor drives.
“We hope to eventually have a self-sufficient CPS that would not require external power, computation and network resources,” Moon said. “Eventually we will have a tiny sensor node (CPS) that could notify you of an anomaly, without the worry of maintaining a battery or other power source as well as requiring data processors and existing network infrastructure.”
For more information about this research at IEEE.